Webinar: Releasing ICU Bed Capacity Using Simulation
London Health Sciences Centre and Ivey Business School utilized Simul8 healthcare simulation software to evaluate the implementation of ‘step-down’ or 'level 2' beds, identifying positive impacts on ICU patient throughput, length of stay and costs.
In this webinar, Dr Claudio Martin from London Health Sciences Centre and Felipe Rodrigues from Ivey Business School discuss how their research team used discrete event simulation model for Planning Level 2 ‘Step down’ Bed Needs Using NEMS.
About the webinar
In highly congested hospitals it can be common for patients to overstay at Intensive Care Units (ICU) due to blockages and imbalances in capacity.
Step-down beds (also known as Level 2 beds) have become an increasingly popular method of avoiding unnecessary ICU stays. Learn how the team at Ivey Business School used simulation to predict Level 2 bed needs for London Health Sciences Centre (LHSC), a large university hospital in Canada.
In this webinar, Dr Claudio Martin from London Health Sciences Centre and Felipe Rodrigues, researcher from Ivey Business School discuss how their research team used discrete event simulation model for Planning Level 2 ‘Step down’ Bed Needs Using NEMS.
Watch the webinar to learn more about how simulation was an essential part of the research to evaluate the needs of step-down beds to maximize ICU patient throughput, deliver efficiency and minimize cost.
Webinar highlights:
- Introduction to the research (4:00)
- Challenges (8:30)
- Modelling scenarios (23:00)
- Research conclusions (38:00)
- Q & A (42:00)
Webinar transcript
Introduction
Thank you. My name is Felipe Rodrigues and I am speaking from London, Ontario. I am a researcher for Ivey International Centre for Health Innovation and with me are my colleagues, Dr Claudio Martin, former chair of the ICU at London Health Sciences Centre, and also Judy Kojlak; she is a director of Clinical Redesign for LHSC.
Thank you everyone for joining us. I will be presenting the research [00:30] that we conducted together, using Simul8, and it is called the Discrete Event Simulation Model for planning level 2 step-down beds using NEMS. This research was conducted by myself, Dr Greg Zaric, Dr David Stanford and also Judy Kojlak, Fran Priestap and Dr Claudio Martin. We are from Ivey Business School at Western University [01:00] and also the Statistics Department at LHSC.
Ivey International Centre for Health Innovation is one of the supporters of [inaudible] research, so I would like to request Dr Claudio Martin to give a quick introduction to LHSC and then we can resume with the programmes [01:30] and how we approach our research.
Read the full transcriptDr Claudio Martin, London Health Sciences Centre
Thanks, Felipe. To put the coming presentation into a little bit of context, as you have heard we are from the other London, in Canada, in the province of Ontario. London itself is about 250,000 population. We are a tertiary and quaternary referral centre with a catchment area of approximately 1 million and the province of Ontario has a population of about 10 million. [02:00] Felipe referred to NEMS – the nine equivalents of nursing manpower score, which is a metric that you may be familiar with, as a nursing workload tool looking at ICU-type interventions. Every ICU within the province of Ontario collects and submits that as part of a province-wide standard dataset for all level 2 and level 3 ICUs. In our centre, London Health Sciences Centre, [02:30] we have two major medical, surgical ICUs and a cardiac unit, totalling around 69 beds between those three units.
We do not have any general level 2 units. That is the jargon we use in Ontario; in other places they may be known as step-down units or high dependency units. We have some service-specific step down [03:00] or level 2, units and what we were interested in was looking at opportunities for improving patient flow through the hospital, which I am sure all of you experience challenges with, to see if there was a possibility to improve patient flow and be more efficient through a redesign of our level 3 and level 2 units, and that is how we came to be involved with Felipe’s project, to try to model [03:30] what this might look like for us in the future. That is a little bit of context for the work that Felipe undertook with us. Thank you.
Discrete Event Simulation Model for Planning Level 2 ‘Step down’ Bed Needs Using NEMS
I. Levels of Care
As Dr Claudio said, we will be dealing with the medical surgical intensive care unit, the MSICU, and the particular metric we are using is the NEM score: the nine equivalents of nursing manpower Score. [04:00] This is generated from another metric called TISS, which people may also be familiar with, but the rule of thumb here is that this is a 56-point scale that measures the nursing workload, and we are going to be using that to define step-down rules for our problem. In short – more definitions here – we have those three levels of care. [04:30] The last row of this table shows that the level of care 3, that is intensive care beds. Usually there is ventilation, patient-to-nurse ratio is usually one-to-one, and these are very costly beds and patients in ICU at level 3 level of care usually have a daily NEM score and they are usually between 26 and 56 points on that particular score. [05:00]
Then we have what would be the step-down beds. Usually those are non-ventilated patients. The cost goes down significantly and the NEM scores are anywhere between 11 and 25.
You finally have the standard ward beds, level 1 care, where you have three or more patients per nurse, maybe five or so. Those are cheaper beds and the NEM scores are the lowest.
With all that in mind, [05:30] when we got together to do this research, the university hospital site was the one picked to conduct this experiment. We had a data set regarding 2014 where we had over 17,000 patients, and out of all of those in patients to the hospital we had roughly 42,000 internal movements of patients inside the hospital. [06:00] The particular thing that came to our attention was the fact that off-service – meaning the misplacement of patients into wards which they are not originally supposed to go – would range anywhere between 5% and 30%. Canadian hospitals tend to be busier than hospitals in the US, for example, so it is quite common that you would find congestion [06:30] and blockage downstream, specifically in the wards, so it ends up with the MSICU, out of 864[?] patients in that year, roughly 666 were eventually measured with a NEM score that would enable them to step down into a level 2 ward, releasing those ICU beds.
II. Step-down Units
The idea behind the research [07:00] was: if we know that these patients may no longer need ICU care, and that they could step down, how do we solve this problem? The LHSC had the plan to add a step-down unit. At first, what we wanted to do was see what the benefit of that one particular step down unit was, coming from the MSICU, [07:30] in terms of patient flow, in terms of throughput, and whether there was a reduction in terms of length of stay and costs. Particularly for UH, how many level 2 beds should be needed and how efficient can those beds be? Finally, since we have those daily measurements of NEM scoring, would that be a useful tool to estimate the moment [08:00] of patient step-down and therefore aiding in the estimation of the level 2 bed needs?
III. Challenges
Figuring out what that one particular unit should be like, in terms of capacity and patient flow, was a big challenge, not only because of the complexity in terms of the care of ICU patients, but also because UH [08:30] has no particular history of medicine level 2 wards, so we had to come up with different rules in order to establish what would be a level 2 patient. The hospital also has a lot of seasonality in terms of patient flow and patients come from many different diagnostics and origins, so heterogeneity is a big issue here.
One thing that we noticed [09:00] was that patient’s health and death probability change over time. So, when we looked into the data we figured that the odds of a patient’s survival being increased as they spend more time in the ICU, and also the way their health progresses changes over time. It was quite clear from a modelling perspective [09:30] that we could not do anything very simple and that we had to incorporate the patient’s health progress and death probability into our simulation.
Some other challenges that we faced were that not only were we considering step-downs, as you progress through the hospital you enter the emergency department, the operating room, ICU, and then eventually step-down wards and so forth, [10:00] but there is a lot of bounce-back of patients coming from the wards, back into ICUs and operating rooms. The hospital is very congested, so that leads to off-service, and an increase of costs and length of stays and so forth. So, when we first met the LHSC measurements, we figured we could have an analytical model to deal with this.
But given all these characteristics [10:30] it was quite clear that we needed a discrete events simulation model, so that is when we decided to come up with a discrete events simulation model that had, in effect, three objectives: the initial one would be to try to maximise patient throughput, while minimising the length of stay in the ICU, and later on we added [11:00] the idea of trying to minimise cost to the hospital.
Our decision variables would be the number of level 2 beds and the number of MSICU beds in our simulation, so as we increase or decrease the number of these two kinds of beds in their respective units, what changes in terms of throughput and patient flow and so forth?
There are quite a few parameters that we had to consider, [11:30] including the arrival rates. We are taking about patients coming from ER, coming from the operating room and so forth, and it changes over time and the days of the week, hour of the day, so that is actually quite complex. There is the length of stay in each one of the units that we are simulating in the hospital, and the death rates, or the survival odds of patients in each one of those units. [12:00] We also had to take into consideration the NEM scoring process. NEMS is collected daily, so we had to figure out what exactly is the threshold that we would consider for the step-down and what would remain in the ICU and how that changes over time.
We also had to pick a time and place in the hospital where the beds allocated to each unit were roughly the same [12:30] so we could consider the changes in patient flow in due order. We had to come up with a patient flow matrix to know where the patients are going to and coming from and finally what kind of budget we would be dealing with when we were considering the simulation and the baseline scenarios and so forth.
IV. Data
In order to do the research, we had to collect data from multiple [13:00] different sources. Gladly, LHSC has a very comprehensive patient management system. So, not only did we have patient information in terms of when they arrived and patient characteristics, but we also had very precise patient transfer files. We actually knew the level of the individual beds where the patients came to and from in each unit of the hospital. The nursing workloads [13:30] as NEMS is recorded at least upon arrival and once daily, and when the patient leaves the unit, so we had a very comprehensive data file showing the NEM scoring process for each one of the patients that went into the ICUs and with all that information we were able to do a very lengthy and precise process of distribution fittings in terms of the arrival rates of patients into the hospital, [14:00] the individual lengths of stay in each one of the hospital units and the probability of step-downs.
With that we created the patient flow matrix and the step-down rules to fit into the simulation. Overall, we had 17,380 patients that we had complete records from and out of those we created all the parameters for the simulation. [14:30]
V. Patient Flow
If I could simplify the patient flow in the hospital, this is what it would look like. We have several entry points that are quite common to a lot of hospitals, such as the emergency department, the operating room, but also several different clinics in town. Also, our sister hospital Victoria Hospital is over here, and transfers from different hospitals in the region. So, when patients come into the hospital, they come in through these entry [15:00] points and from there they can go to any one of those three levels of care – the level 3, level 2 and level 1 being the wards. So, when I first approached this research I had the idea that patient flow would be quite linear and flow downstream. What we find is that actually patients go to and from all of these places. So, the transition matrix is actually very complex [15:30] and we had to address that to a very high level of detail. Currently there are two level 3 units, the CSRU and the MSICU; we were focusing particularly on MSICU patients. The current level 2 units are very specialised for transplant and neurology and CCU care, while the different wards have their own kinds of patients. [16:00] So, if we can translate this one chart into a flow matrix this is what it looks like. So, from the patient transfer files we were able to figure out exactly what the odds are of patients going to and from everywhere.
So, for example since we are talking about MSICU, this one particular intensive care unit, 8.6% of patients are routed into operating rooms, [16:30] 16% of those patients, being the bottom centre of the chart, they eventually go into the fourth floor and 22% of the patients, unfortunately, end up dying. We were able to feed in all of this information to the simulation and see how the simulation would perform.
This is a sample screen of our model, [17:00] so like I showed before we have several different entry points. The emergency room here to the top left, we have Victoria hospital here on the bottom left corner, the operating room here also in the bottom left corner. Different ICUs, level 2s and wards, and patients can eventually die, be discharged, or transferred to different hospitals. If you allow me, I will show the model running for a little while. [17:30] This is a static screenshot; let me see if I can hopefully show a little bit of the simulation working.
This is just a sample simulation of what we did. As you can see, over here, we have patients coming from the ED and operating room and they are being transferred to any of those units. [18:00] So, let me speed this up a little bit. We can see that the simulation is populating the hospital. Pay particular attention to the centre part of the simulation, where we see the MSICU patients as they go up and down in time. And then we have several different key performance measurements that we are tracking such as the number of patients [18:30] in the level 2 units, the number of patients in the MSICU and so forth. If I speed this up a little bit more then we can see how things change over time. So, I am simulating two years’ worth of simulation here. So, we actually have quite a lot of data collected from the simulation to a very high level of detail. You can see as things change over time, the hospital becomes busier [19:00] and you see how seasonality affects things such as operations only happening on weekdays and so forth, how all those things interfere with the occupancy of the hospital and the different units. Even though we are talking about patients in MSICU stepping down into a level 2 unit, because of all the congestion that happens in the hospital in the off-service [19:30] that we encounter, we had no alternative but to simulate the entirety of the hospital patient flow and we can see why that was wise as we were able to track the impact of the level 2 unit in terms of off-service as well. If we had just picked this one particular part of the simulation, just the ICU [20:00] and the level 2 units, we would not be able to capture blockage and congestion and off-service. So, even though it took longer to perform the simulation, it was the wider decision to make as we had a wealth of data that we could analyse and make decisions on.
VI. Death Probability
Going back into the presentation, one of the things that we noticed for the MSICU is that [20:30] the death probability changes over time, as does the probability of stepping down. So, we had to come up with a system that would mimic what actually happens in the ICU. The first thing that we noticed is that we had to change the paradigm a little bit. We considered the MSICU as a queue [21:00] as opposed to a service and what happens is, once the patient arrives into the MSICU, the simulation allows us to divide that patient into two different parts. One being the physical patient in the MSICU, so they are occupying a place in the queue here in the MSICU, so that would be a bed, and the other half [21:30] of the patient, as we call it the procedural part of the patient, goes into a stochastic model of death and NEM scoring. So, that one patient waits about 24 hours and then we sample the death probability of that one patient. If the patient survives that 24 hours, the patient is then taken into the NEMS measurement [22:00] and for instance if that one particular measurement is enough for the patient to step down, then the procedural patient is joined and then leaves the ICU. But if the patient is sampled with a NEM score that says this is a level 3 patient and so it has to remain in the ICU, then the patient returns into the death process, waits another 24 hours, and then is sampled a death probability again. [22:30] In this one particular example, the patient might actually be sampled to die and then, unfortunately, the physical patient and the procedural patient are joined again, and collected and they are taken out of the simulation as a deceased patient.
VII. Modelling Scenarios
Doing the stochastic modelling enabled us to see exactly how the length of stay of the patient is not deterministic; [23:00] it is actually a function of the death and NEMS scoring that happens over time. What we are basically saying is that the length of stay is a function of how the health of that one patient progresses over time. That enabled us to do many different scenarios, the first one being the ideal world where we have unlimited money and real estate [23:30] so we can add new capacity from anywhere from two to 20 beds, into a new level 3 unit, while keeping the existing MSICU beds as they are. The second scenario would be re-allocation of existing capacity. So, there are currently 25 beds in MSICU, so let us say we convert some of those beds into level 2 beds and see how that re-allocation performs in the hospital. [24:00] And the third scenario is when we add five extra beds, so from 25 we go to 30 beds and we allocate them between the MSICU and a new level 2 unit.
Now let us go into some model validation. We performed the simulation first with the baseline scenario, [24:30] to ensure that the simulation was realistic with time and what we saw in real life. So, for any comparison matters, throughput was roughly the same, length of stay was actually very accurate, also the cost was very accurate. The eventual MSICU length of stay was actually quite close to what you see in the empirical data, maybe a little bit higher albeit with a [25:00] smaller standard deviation. The simulation could not quite make into the long tail that you find in lengths of stay in ICU patient, but statistically it was close enough that we could take it on and every other validation measurement that we found showed that the baseline model worked fine [25:30] so we could then go into different scenarios.
VIII. Off-Service Impacts
The first one that I would like to show is the impact in terms of off-service. In the baseline scenario we had, with the existing level of level 3 and 2 units, they had roughly an impact of 600 patients in terms of off-service. This could be patients that could eventually go into wards that they are not supposed to go to, but they had to due to congestion. [26:00] As you can see from different scenarios, scenario one a different capacity, scenario two re-allocation and scenario three the added extra beds with re-allocation, you can see that off-service decreases a great deal once you add the level 2 unit. Then we could also see in terms of throughput what happens in the hospital with both the MSICU and the level 3 unit. [26:30]
This chart shows in the x axis the number of level 2 beds that we are adding into the scenarios and the y axis is the throughput of patients per year. So, if you follow the blue lines, this is MSICU throughput. Scenario one stabilises roughly after eight beds or so, while scenario two, where we are re-allocating beds, there is an increase in throughput and then you see [27:00] this concave behaviour as the number of MSICU beds decrease then patient flow is constrained and throughput drops quite sharply. When we add beds into the third scenario then you can see we have a broad range of scenarios, in terms of level 2 beds, where throughput is roughly the same. Anywhere between 8 and maybe 16-18 beds, [27:30] in terms of level 2 beds, would suffice in terms of throughput and you can see a similar behaviour happening in terms of level 2 beds.
So, the first scenario stabilises roughly after eight or 10 beds, the second scenario there is a concavity you find around 12 beds and third scenario you have a broader range in terms of throughput. Regarding utilisation rates, you want to have empty beds in case of a surge of patients, [28:00] but you do not want to have too many empty beds. So, in this chart we show in the x axis the number of level 2 beds in the simulation and the utilisation rate on the y axis and you can see the blue lines. First scenario, utilisation rates drop dramatically and they keep falling up to 30% utilisation rate, that means there are many empty beds in the end. [28:30] The second scenario you see the convexity that we saw earlier roughly around 10 beds, where you reach the minimum utilisation. And then for the second scenario you see around eight or 10 beds, which is the minimum utilisation rate you can get. So, by adding those beds, you see that there is great gain in terms of empty beds and capacity to accommodate surge of patients throughout the year. [29:00]
This chart shows the number of empty beds in the y axis. Basically, instead of talking terms of utilisation rates we are talking about absolute numbers of empty beds. You can see that up to a point when you add all those beds into the hospital, you eventually reach up to 17 or so empty beds [29:30] on average in scenario one. That is too many. That is unused idle capacity, which you do not want to have. You want to make sure your capacity is being well utilised. You can reach up to 18 empty beds while today we have almost no idle capacity at all.
IX. Length of Stay
In terms of length of stay, that was a question we had to address. [30:00] As you increase the number of level 2 beds in the x axis, you see that the length of stay drops dramatically. We go from the original 160 hours or so, as you start adding level 2 beds, in the beginning there is a sharp increase and then as you start unconstraining the patient flow into the level 2 unit there is a sharp drop and eventually [30:30] you reach the threshold of roughly 60 hours. Those 60 hours, out of the 160, means a lot of extra capacity that you add into the level 2 unit as you release those ICU beds to receive new patients from emerge or from the operating room. The scenarios where you are reallocating beds, [31:00] eventually as the level 2 unit is bottlenecked by the MSICU, then the length of stay starts to increase a little bit.
An unintended finding from our research was that as you have a certain number of long-stay patients in the MSICU, these are patients [31:30] who happen to be in ICU care for more than 21 days, or roughly 500 hours, as you add level 2 beds, the stochastic process of NEM scoring eventually releases almost all of these patients into the level 2 unit. While we have in the baseline scenario something like five or six per cent of long stay patients, we see that the NEM scoring process eventually leads them [32:00] to a level 2 NEM score, so they are all stepping down as you add level 2 beds. This happens around six or eight beds also, for all three scenarios.
X. Cost Savings
This one particular chart is, for me, what I would call the cherry on the cake. As we added estimated operational costs into the simulation – and from an analytical perspective [32:30] this was great because you have this nice convexity here where you can find minimal points – for all three scenarios we go from a daily cost of roughly $3,500 in the MSICU. As you add level 2 beds in the x axis, you see that the cost per patient/day drops dramatically reaching a minimum around 12 beds [33:00] for all the scenarios. Up to 12 beds there is a significant gain in terms of economies of scale that you get for adding the level 2 unit and from those 12 beds over, you actually start having idle capacity of some sort and the patient/day cost starts to increase as the MSICU is reduced in size and eventually you end up [33:30] having a cost increase that could lead back into the original $3,500 cost per patient/day. This gives a nice threshold of anywhere between 10, 14, maybe 16 beds in terms of level 2 beds in the end, answering one of those questions.
XI. Alternative Scenarios
Another thing that we wanted to see was that this works well into our current scenarios, [34:00] but what if we increase patient flow? We tried all sorts of scenarios in terms of patient flow increase, going from 5% all the way to 30%. This is an example of the first scenario where we add 12 beds into the system, while keeping the same number of ICU beds. We can see that the number of patients waiting for a position in ED, we do not want those patients to wait [34:40] too long for this position, so anywhere below five minutes is fine, so in the baseline and this one scenario we are roughly at 99%. As you can see as patients increase, patients start to wait too long. Two hours, 26 hours, so we see that there is a threshold where the hospital basically starts to collapse anywhere between 10 and 20% in terms of increase, [35:00] but adding those level 2 beds helps stabilise that one particular influx of patients.
Throughput, so this is a chart where we had a bed mix. We have 30 beds available and we are reallocating MSICU beds into level 2 beds, you see the original scenario here in the bottom, blue and orange. We had a nice long threshold where different range of beds [35:00] would suit our needs. As you can see the patient flow increases we lose that wiggle room and we start converging into 16 MSICU beds and 14 level 2 beds in the end. So, things start to be much more responsive and there is a lot less room for patient surges.
The average length of stay [36:00] up into maybe 10% increase of patients, we roughly do not see any change in terms of average length of stay in the ICU, but then we see an increase in terms of hours in patient stay. So, 30% increase, that is already a big deal in terms of adding another 5 hours into another 1000 or so patients, so a much higher level of occupancy and things start to deteriorate. [36:30]
Finally, in terms of sensitivity analysis, when we see our total bed cost, when we account the operational cost for the MSICU and the new level 2 unit, you see in the original scenarios where we had the 30-bed capacity reallocated among those two units, you can see that there is a range of bed mixes that are roughly around [37:00] the $15 million mark and as you increase patient flow we lose that ability to deal with the range of patients. So, if we wanted to make something like a dynamic setting for those two units, we would lose that ability as patient flow increases and then we would start converging into a different costing structure, going all the way up to $20 million in terms of operational cost. [37:30]
XII. Conclusions
In summary, what we find, is comparing to the baseline, we have three different scenarios where we can find optimal points and adding the level 2 unit seems to converge around 12 beds out of the initial 25 beds in MSICU. Scenario one would have too many beds available, 37 beds that is too many, so we can actually [38:00] go into different scenarios, such as scenario two or three, where we are reallocating beds, or adding just a few more beds, from 35 to 30 beds in total, and we can see that there is a sharp drop in terms of average utilisation rates, while we can still account for a surge in patient influx. That means we can reduce the off-service in the ICUs. [38:30] We can also see that the length of stay is sharply reduced and, particularly in terms of cost, we have minimal points that are significantly lower than what you would expect from the baseline scenario. Again, these are cost estimates, so we are talking about rough numbers here, but you can see that there is room enough to reduce by many millions of dollars the current operational cost of that structure [39:00], and this is something that only a very precise and detailed simulation could provide results for.
In terms of conclusions, I would like to say first of all there seems to be a clear positive impact, especially when we simulate the new level 2 unit into the current patient flow. We reduce occupancy, utilisation rates go down, length of stay of MSICU patients go down, [39:30] as well as off-service and cost. So, it is clear that this could have a significant impact in terms of patient flow. Adding capacity, there is a moment where that does not benefit the hospital anymore, so we have to be careful not to overshoot, or undershoot. There is a sweet spot that we can achieve and it seems that converting MSICU beds into level 2 beds would have some benefit, particularly [40:00] in case of budget constraints and so forth.
In the end, adding the NEM scoring process and the stochastic death probability process into the simulation proved to be very useful and very precise. This is something that I would strongly recommend if anyone is simulating ICUs, make sure that you have the death probability changing over time and the NEMS scoring process, [40:30] meaning the health of the patient, changing stochastically. That seems to provide very reliable results, so I would recommend ICU simulations to consider those two aspects of patient health. Some of the next steps that I am conducting and we have started to talk about would be perhaps adding new simulations into the sister hospital, [41:00] which is Victoria Hospital, or having simulations where we have dedicated long-stay beds, maybe Dr Claudio can talk a little bit more about that later on.
Two projects that I am conducting on the side is to come up with a forecasting model to see the length of stay in the MSICU using those NEMS measurements that seem to behave so well in the simulation, and also see if I can simplify this a little bit and perhaps use an analytical model [41:30] that would incorporate the off-service, the NEMS measurements and the bounce-backs that we see in the patient flow and use the simulation to validate that analytical model.
This is what I have to say; maybe Dr Claudio and Judy would like to add a little bit more about their experience with using the simulation.
Questions and Answers
Moderator
[42:00] Felipe, thank you so much for that very comprehensive and fascinating explanation about the simulation. I do not know if colleagues from London Health Science Centre are willing to say anything or wanted to comment anything further on this about what is likely to happen as a result?
Dr Claudio Martin
[42:30] We worked a fair bit with Felipe on this model and there was a fairly rich amount of data to feed into the model which he did describe. We are moving ahead making some changes, it is an ongoing project. The results from the simulation are just one tool, or one piece of data being used by the team [43:00] to consider how to redesign our overall bed model within the hospital. There are other constraints, physical, client and so on, that need to be considered but results from the simulation were definitely very helpful and consistent with our conclusions from some other thought processes as well. It has been a very useful project [43:30] to be involved with on a very practical point.
Moderator
Thank you very much. It is really good to hear that it is having an impact. There are a couple of questions that have been posted if you are okay to answer those. I will read the first one. This is from someone completing a PhD in simulation and modelling at the University of Saskatchewan saying, ‘Fascinating research and an excellent presentation, thank you. [44:00] Do you plan on running scenarios where you change the staffing mix?’ So, either looking at RN versus RPN versus care aids, or changing the percentage looking at financial impact. Can you say anything about that?
Felipe Rodrigues
So, this one particular model the focus was in terms of how many beds we should have and keeping in mind that patient nurse ratio is an important aspect of both the patient flow and cost. [44:30] The idea was to figure out the number of beds. Now, adding staffing into the model should be another layer in the model that we could certainly do. I think as we simulated the entirety of the hospital one could have perhaps a very bold approach and say, [45:00] ‘Let us have staffing all over the hospital and see how the hospital behaves in terms of nursing and so forth.’ Or one could just go into the MSICU and the level 2 units and add nurses there, or different kinds of health professionals and see their occupancy and their idle time and so forth. We did not intend to do that at first, but it can be done in this simulation.
Judy Kojlak
It is Judy Kojlak, from LHSC. [45:30] I think it is fair to say that the skill mix initiative is a current corporate initiative that is underway right now. Right now, based on the acuity measures and those patients that are admitted and occupying the level 3 beds, not so much the level 2 beds but the level 3 beds, right now the information would suggest [46:00] that we do not have capacity in terms of moving in an RN, RPN skill mix. But again, that is a corporate-wide initiative and there are new tools that we are using in terms of assessing the acuity indexes of patients occupying beds in level 2, level 3 and the wards. But the primary function and focus right now is focusing on [46:30] skill mix in the level 2 and on the ward.
Moderator
Okay, thank you very much for that. A couple of other questions that have come in. So, based on your simulation, what was the time span of the data you estimated?
Felipe Rodrigues
We had data worth of about a year of patients and that was the minimum that we needed [47:00] because there is so much seasonality involved. Not only did it have to be a year, but it also had to be in a year where we had a fixed number of beds in the units so that data would be precise to what we were trying to simulate. When we simulate this, again, we run the simulation for a couple of years warm up time and then we collect data for another year. And we do that hundreds and hundreds of times, [47:30] so there are thousands of hours of simulation in our results, but because of seasonality I would recommend at least a year’s worth of data and a very long warm up time, because the patient flow is so complex that you have to allow for the hospital to be fully populated and I found that one year was not enough, two years was good to stabilise the simulation, [48:00] and then collect data for another year. Multiple, multiple times.
Moderator
Okay, that is really interesting. And did you include factors like the aging of the population?
Felipe Rodrigues
No. There is a host of characteristics that we could have included in the simulation, but when we were looking into the hazard rates for the MSICU patients, [48:30] in terms of their odds of survival overtime as they change, we were able to find a good enough fit, log normal distribution taking into consideration time, and that was sufficient for the simulation to run to realistic levels. Not only patient age, but also diagnostics and so forth, [49:00] all of these things play a role in the patient’s probability of death and probability to step down. So, you could dig into those kinds of issues but we found that accounting for time in that one particular population group, that was enough of a fit to run the stochastic simulation that way.
Moderator
Thank you so much to everybody. [49:30] Look forward to talking to you again as a future webinar, and particular thank to Felipe and colleagues from London Health Science Centre.
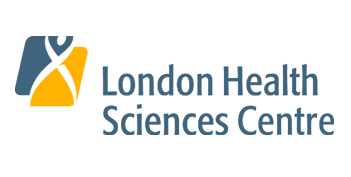
"The results from the simulation were very helpful and provided additional evidence to reinforce our decision-making. It has been a very useful project to be involved with on a very relevant question."
London Health Sciences Centre
London Health Sciences Centre projected $10 million of potential cost savings over span of 8 years using simulation
Read the case studySubscribe to our newsletter
Hear about our latest software updates, user stories, Simul8 tips and more - straight to your inbox.